Last Updated:
11 February 2025
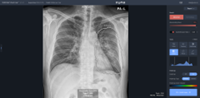
VUNO Med-Chest X-ray accurately detects and flags suspected chest abnormalities indicative of major pulmonary diseases from chest X-ray images. The solution provides information on findings of chest related abnormalities, abnormality scores as well as their locations, maximizing the reading accuracy and efficiency of radiological reporting. Pleural Effusion and Pneumothorax demand exceptional performance in emergency situations. Our solution offers FDA-approved, top-tier performance. AI measures the ratio of the heart to the thorax, providing the Cardiothoracic Ratio to help predict cardiomegaly. VUNO Med Chest X-ray enables integration with various clinical workflows. Our lightweight solution allows for easy use in hospital settings without the need for server infrastructure.
Certification
CE MDD v1.1, CE MDR v1.2(2025.02), Korea MFDS v1.2, Thai FDA v1.2, Indonesia FDA v1.2, Malaysia FDA v1.2
Development Stage
On the Market
Deployment
Online & Offline
Intended Age Group
19+ years
Target Setting
Primary health centers, general hospital (above primary level), teleradiology companies, government/public sector, e.g. national TB programme, private sector
Current Market
Asia, Europe
Input
Can be used to read images from any kind of digital X-ray machine and model.
Chest X-ray image format: DICOM
Chest X-ray type: Posterior-Anterior CXR, Anterior-Posterior CXR.
Output
Output includes:
Heatmap
Dichotomous output indicating whether TB is likely present or absent
Dichotomous output indicating whether each abnormality is likely present or absent
Probability score for TB
Probability score for each abnormality
Location of each abnormality
Statistics: this feature provides statistical data on detected findings monthly and allows user to analyze frequency and trends using graphs on a monthly basis.
If nodules or consolidations are suspected to be TB, the TB assessment utilizes the abnormality score of these findings. The adjustment feature is currently under development and will be available in the future.
Reports provide detailed descriptions of each abnormality and location using a familiar hospital PDF template. Additionally, AI interpretation results are provided as a text report.
Lung abnormalities included in the TB Score: Consolidation, Mass, Nodules
Additional findings reported by the product: Consolidation, Mass, Nodule, Pleural effusion, Pneumothorax



Hardware
This product operates seamlessly with both CPU and GPU configurations. Compatible with both mini PCs and laptops.
Server
The CPU should be an 8th-Gen Intel Core i5 processor or higher, and the GPU should be a GeForce RTX 2070 with 8GB RAM or more.
Integration with X-ray Systems
Integration with PACS and Legacy Systems
VUNO Med-Chest X-ray has been tested with global X-ray imaging equipment from brands such as GE, SIEMENS, PHILIPS, SAMSUNG, FUJI, and CANON. It seamlessly integrates with any X-ray device, connecting via the DICOM protocol, and can also be integrated through an API.
VUNO Med-Chest X-ray seamlessly integrates with various PACS using DICOM C-Store. This product can be effortlessly incorporated into the client's existing storage and transmission systems.
Software
Ubuntu 22.04, Windows 10, or Windows 11, minimum 8 GB RAM, minimum 8 GB GRAM (if using GPU)
Processing Time
5-20 seconds
Data Sharing & Privacy
This product uses SSL/TLS to ensure data integrity and confidentiality, thereby protecting it from attacks. Plus, AI results and original data can be configured not to be stored in the database. After results are sent to PACS, and all data is deleted if such option is configured.
Software Updates
VUNO Med-Chest X-ray is updated at least twice a year, ensuring regular product enhancements. Customers are informed of the update details to keep them well-informed.
Product Development Method
The algorithm for AI analysis is a model trained using a reference standard from the training data, based on a type of deep learning called Deep Convolutional Neural Networks (CNN). This deep learning technology is a machine learning algorithm that autonomously learns the features of each data point.
Training
VUNO Med-Chest X-ray has been trained on approximately 150,000 chest X-ray images for 19 years or older individuals acquired from multiple institutions across Korea, the United States, and Europe.
Reference Standard
The reference standard is established using CT-confirmed data, meticulously labeled by two experienced radiologists.
Publications
1. Sung, J., Park, S., Lee, S.M. et al. Added Value of Deep Learning-based Detection System for Multiple Major Findings on Chest Radiographs: A Randomized Crossover Study. Radiology. 2021;299(2):450-459. https://doi.org/10.1148/radiol.2021202818
2. Kim, YG., Cho, Y., Wu, CJ. et al. Short-term Reproducibility of Pulmonary Nodule and Mass Detection in Chest Radiographs: Comparison among Radiologists and Four Different Computer-Aided Detections with Convolutional Neural Net. Sci Rep. 2019;9:18738. https://doi.org/10.1038/s41598-019-55373-7
3. Park, S., Lee, S.M., Lee, K.H. et al. Deep learning-based detection system for multiclass lesions on chest radiographs: comparison with observer readings. Eur Radiol. 2020;30:1359–1368. https://doi.org/10.1007/s00330-019-06532-x
4. Kim, W., Lee, S.M., Kim, J.I., Ahn, Y., Park, S., Choe, J., Seo, J.B. et al. Utility of a Deep Learning Algorithm for Detection of Reticular Opacity on Chest Radiography in Patients With Interstitial Lung Disease. AJR. American Journal of Roentgenology, 2021;218(4):642-650. https://doi.org/10.2214/AJR.21.26682
5. Park B, Chung Y, Jung KH, Seo JB et al. Utilizing Synthetic Nodules for Improving Nodule Detection in Chest Radiographs: Journal of Digital Imaging, 18 Mar 2022, 35(4):1061-1068. https://doi.org/10.1007/s10278-022-00608-9
6. Kim HW, Ko HB, Kim JJ. et al. PILLET-GAN: Pixel-Level Lesion Traversal Generative Adversarial Network for Pneumonia Localization: Proceedings of the 5th International Conference on Medical Imaging with Deep Learning, PMLR 172:676-688, 2022. https://proceedings.mlr.press/v172/kim22a.html
7. Lee JH, Ye SY et al. A Comparative Study of Deep Learning Models for Pneumonia Detection: CNN, VUNO, LUIT Models. Vol. 18, No. 3, pp. 177-182, 2024 https://doi.org/10.23042/radin.2024.18.3.177.
This website works best with browsers other than Internet Explorer.